Understanding Soil Health
Key Indicators of Soil Health
Soy health is critical for sustainable agriculture.
It encompasses biological, chemical, and physical properties.
Key indicators include soil organic matter, pH, and nutrient levels.
Soil organic matter influences its structure and fertility.
pH affects nutrient availability for plants.
Nutrient levels determine the productivity of crops.
Metrics for Measuring Soil Health
Several metrics help quantify soil health effectively.
These metrics provide insights into soil conditions.
Common metrics include soil respiration and microbial biomass.
Soil respiration indicates biological activity and health.
Microbial biomass illustrates the community of soil organisms.
Incorporating these metrics enhances data-driven decisions.
The Role of Data in Assessing Soil Health
Data collection plays a vital role in understanding soil dynamics.
Technologies like soil sensors and satellite imagery provide valuable insights.
These tools identify variations within fields.
Transform Your Agribusiness
Unlock your farm's potential with expert advice tailored to your needs. Get actionable steps that drive real results.
Get StartedMoreover, they track changes over time, improving management practices.
Data empowers farmers to make informed decisions.
Ultimately, this enhances overall soil health.
The Role of Data Analytics in Soil Health Assessment
Introduction to Soil Health
Soil health is vital for sustainable agriculture.
It impacts food security, environmental quality, and ecosystem sustainability.
Applying data analytics enhances our understanding of soil health.
Understanding Data Analytics
Data analytics involves collecting and analyzing data to derive insights.
This process enables farmers to make informed decisions.
Therefore, effective data analytics leads to improved soil management.
Types of Data Collected
Farmers can collect various types of data for soil assessment.
- Soil moisture levels
- Nutrient content
- pH levels
- Organic matter content
- Microbial activity
This information helps in understanding soil health comprehensively.
Data-Driven Decision Making
Data analytics allows farmers to make strategic decisions.
For instance, it helps identify areas needing amendments.
Thus, farmers can optimize crop yields and resource use.
Technology Integration
Integrating technology is crucial for effective data analysis.
Soil sensors and drones provide real-time data collection.
Additionally, data visualization tools enhance interpretation.
Consequently, technology streamlines the soil health assessment process.
Case Studies and Success Stories
Many farmers have successfully used data analytics.
For example, Green Fields Farm improved soil fertility using real-time data.
They realized higher crop yields and reduced costs.
Similarly, EcoAgri Services utilized aerial imagery to assess soil health.
This approach led to targeted interventions that boosted productivity.
Challenges in Data Utilization
Despite its benefits, challenges exist in data utilization.
Showcase Your Farming Business
Publish your professional farming services profile on our blog for a one-time fee of $200 and reach a dedicated audience of farmers and agribusiness owners.
Publish Your ProfileData quality and accuracy pose significant concerns.
Moreover, farmers may lack access to advanced technology.
Training is essential for effective data interpretation and application.
The Future of Soil Health Assessment
The future of soil health assessment relies on continuous innovation.
Emerging technologies such as AI and machine learning show promise.
These advancements can provide predictive analytics for soil management.
Ultimately, this leads to healthier soils and sustainable farming practices.
Integrating IoT Sensors for Real-Time Soil Monitoring
Understanding IoT Technology
IoT stands for the Internet of Things.
It connects devices and sensors to the internet.
This technology collects and shares data in real time.
It enables farmers to monitor soil health continuously.
The Role of Sensors in Soil Health
Sensors measure vital soil parameters like moisture and temperature.
They provide immediate feedback on soil conditions.
Farmers can make quick decisions based on accurate data.
This responsiveness improves crop yields significantly.
Types of IoT Sensors
- Soyl Moisture Sensors
- Temperature Sensors
- pH Sensors
- Conductivity Sensors
Each sensor type plays a crucial role in monitoring soil health.
Using a combination of sensors provides comprehensive insights.
Benefits of Real-Time Monitoring
Real-time monitoring enhances resource management.
Farmers can optimize water usage through precise data.
Moreover, it helps in early detection of nutrient deficiencies.
This proactive approach leads to healthier crops and better yields.
Implementation in Agriculture
Adopting IoT technology requires investment in sensors and software.
Farmers need to select the right sensors for their crops.
They can integrate these systems into existing farming practices.
With proper implementation, farmers can significantly boost productivity.
Case Studies of Successful Integration
Various farms have reported success with IoT sensors.
For instance, Green Acres Farm increased yields by 30%.
They utilized moisture sensors to optimize irrigation.
Similarly, Ridgeview Farms achieved greater efficiency.
They implemented pH sensors to monitor soil health closely.
Uncover the Details: Optimizing Water Use with Smart Irrigation Systems
Data-Driven Approaches for Nutrient Management
Understanding Soil Nutrient Needs
Soil health depends on understanding its nutrient needs.
Data analysis helps identify nutrient deficiencies effectively.
Regular soil testing offers crucial insights into nutrient levels.
Moreover, mapping soil types aids in personalized nutrient management.
Utilizing Technology for Nutrient Management
Precision agriculture leverages technology for better outcomes.
Farmers can use drones to assess crop health and soil conditions.
Mobile applications provide real-time data on soil nutrients.
These tools enhance decision-making for nutrient applications.
Implementing Data-Driven Strategies
Data can inform fertilizer application rates accurately.
Showcase Your Farming Business
Publish your professional farming services profile on our blog for a one-time fee of $200 and reach a dedicated audience of farmers and agribusiness owners.
Publish Your ProfileThis minimizes waste and optimizes crop yields significantly.
Farmers should adopt variable rate technology for targeted applications.
Furthermore, integrating data from multiple sources ensures comprehensive monitoring.
Evaluating Outcomes and Adjusting Practices
Regular evaluations enable farmers to adjust their practices accordingly.
Collecting feedback on crop performance informs future nutrient management strategies.
Data analytics can reveal trends over time for continuous improvement.
Adaptation to changing conditions ensures sustainable soil health management.
Learn More: Precision Farming Techniques Using Data
Utilizing Remote Sensing for Soil Quality Mapping
Introduction to Remote Sensing
Remote sensing technology plays a crucial role in agriculture.
It allows farmers to collect data about their fields efficiently.
By using aerial imagery, we can assess soil conditions without physical sampling.
Understanding Soil Quality
Soil quality is vital for sustainable agriculture.
Healthy soil supports plant growth and increases productivity.
Various factors determine soil quality, including texture and nutrient content.
Benefits of Remote Sensing for Soil Mapping
Remote sensing enhances soil quality mapping in several ways.
- It provides high-resolution images of large areas quickly.
- It captures real-time data for timely decision-making.
- It reduces labor costs associated with traditional sampling methods.
Implementing Remote Sensing Techniques
Farmers can use different remote sensing techniques to analyze soil quality.
Satellite-based sensors offer a broad overview of agricultural regions.
Drone technology delivers precise data for targeted analysis.
Case Studies in Remote Sensing Application
Many agricultural firms are successfully using remote sensing.
For example, GreenField Ag utilizes drones for crop health monitoring.
Their data aids in identifying nutrient deficiencies across fields.
Future of Soil Quality Mapping
Advancements in remote sensing will further improve soil mapping.
Integrating AI with remote sensing can enhance data interpretation.
Such technologies will enable precision agriculture for better yields.
Uncover the Details: Implementing Sensor Technology on Your Farm
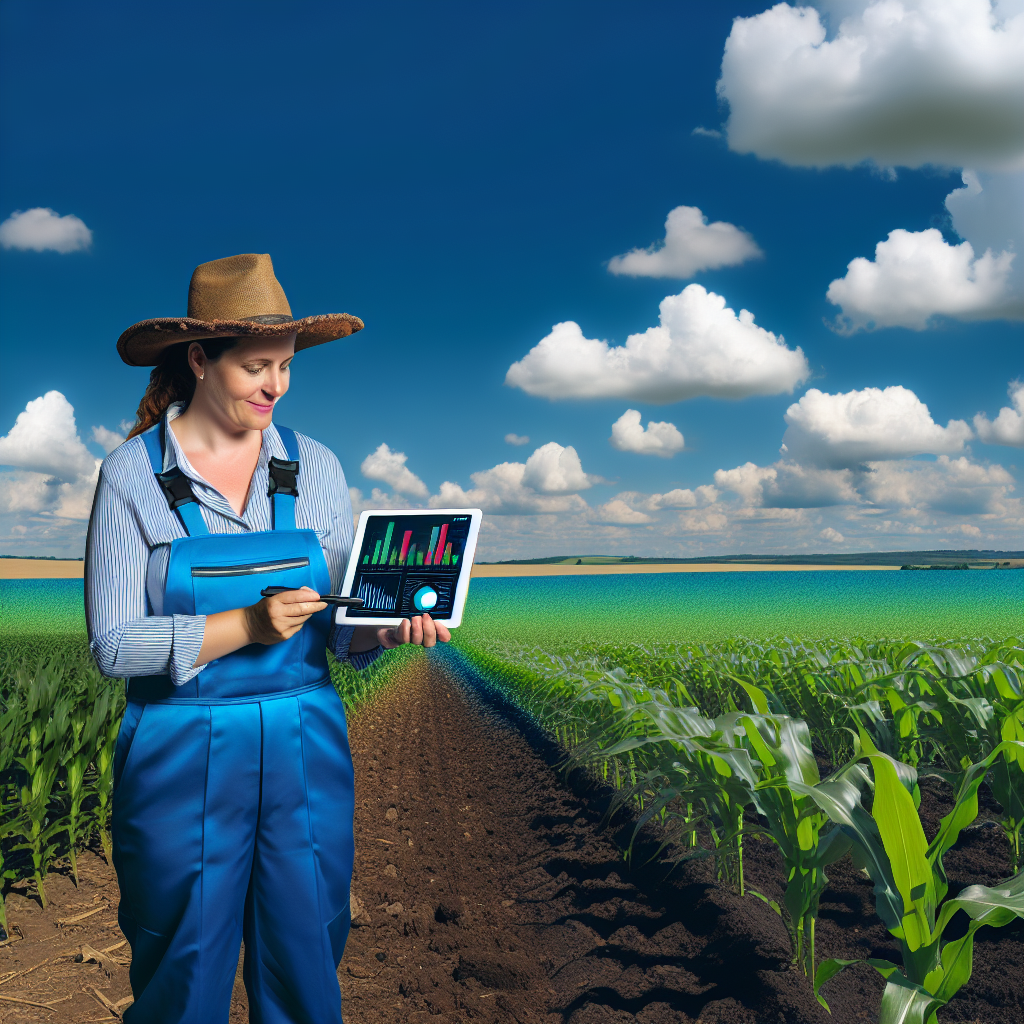
Case Studies: Successful Implementation of Data Insights in Farming
Precision Agriculture at Green Valley Farm
Green Valley Farm adopted precision agriculture to enhance productivity.
They utilized soil sensors to monitor moisture levels efficiently.
This data allowed them to irrigate precisely when needed.
As a result, they reduced water usage by twenty percent.
Moreover, crop yields increased by thirty-five percent in one season.
Data-Driven Decisions at Maple Leaf Orchards
Maple Leaf Orchards implemented a data management system for their crops.
They gathered information on pest populations and weather patterns.
This helped them decide when to apply pesticides accurately.
Consequently, pesticide use decreased by forty percent in five years.
As a bonus, fruit quality markedly improved due to targeted treatments.
Innovative Practices at Cedar Creek Ranch
Cedar Creek Ranch embraced data analytics for their livestock management.
They monitored the health and behavior of their animals closely.
This data enabled timely intervention for sick animals.
As a result, livestock mortality rates dropped by fifty percent.
Additionally, overall productivity increased significantly across the ranch.
Showcase Your Farming Business
Publish your professional farming services profile on our blog for a one-time fee of $200 and reach a dedicated audience of farmers and agribusiness owners.
Publish Your ProfileCollaborative Solutions Through Data Networks
Farmers in the Southfield area formed a data-sharing network.
They shared insights on crop performance and weather forecasts.
This collaboration allowed farmers to make more informed decisions.
Gathering diverse data helped them react swiftly to market changes.
Consequently, their overall profitability improved year-over-year.
Gain More Insights: Implementing Robotics On Your Farm
Machine Learning Techniques for Predicting Soil Health Outcomes
Introduction to Machine Learning in Agriculture
Machine learning enhances agricultural practices through data-driven insights.
This technology predicts soil health outcomes effectively.
Farmers can make informed decisions with this information.
Types of Machine Learning Techniques
Various machine learning techniques offer unique advantages.
Supervised learning applies known data to predict outcomes.
Unsupervised learning uncovers hidden patterns in data.
Supervised Learning
Supervised learning relies on labeled datasets.
It uses historical data to train algorithms for prediction.
Common algorithms include decision trees and support vector machines.
These techniques help in predicting soil nutrient levels effectively.
Unsupervised Learning
Unsupervised learning analyzes unlabeled data.
This method identifies clusters and relationships among data points.
For instance, clustering techniques can group similar soil types.
Thus, it helps researchers understand soil health variability.
Data Collection Techniques
Effective data collection is crucial for machine learning models.
Remote sensing technology provides large-scale soil data.
Soil sensors collect real-time information about soil properties.
Additionally, farmer surveys contribute qualitative insights.
Case Studies of Effective Implementation
Many farms have successfully integrated machine learning.
One study, conducted by GreenFields Agro, used predictive models.
The farm optimized fertilizer use based on soil health forecasts.
This approach resulted in a significant yield increase.
Challenges and Limitations
Despite its benefits, machine learning faces challenges.
Data quality and availability can impact model accuracy.
Moreover, farmers may require training to use these technologies.
Addressing these challenges is essential for broader adoption.
Future Directions
The future of machine learning in agriculture looks promising.
Advancements in technology will improve predictive analytics.
Collaboration between agronomists and data scientists will thrive.
This partnership will enhance soil health management practices.
Best Practices for Data Collection and Management in Agriculture
Importance of Accurate Data Collection
Accurate data collection is essential for farming success.
It helps farmers make informed decisions based on real-time information.
Reliable data enhances understanding of soil health and productivity.
Tools for Effective Data Collection
Utilize technology to streamline the data collection process.
Showcase Your Farming Business
Publish your professional farming services profile on our blog for a one-time fee of $200 and reach a dedicated audience of farmers and agribusiness owners.
Publish Your ProfileDrone technology provides aerial photos for comprehensive analysis.
Soil sensors deliver real-time moisture and nutrient levels.
Mobile applications simplify data entry and management.
Establishing Standard Procedures
Standardized procedures enhance consistency in data collection.
Train staff on best practices for gathering and recording data.
Regularly review procedures to ensure they remain relevant.
Data Storage Solutions
Choose secure and scalable data storage options.
Cloud-based solutions offer easy access and collaboration.
Ensure data backup to prevent loss during technical failures.
Analyzing and Interpreting Data
Analyze collected data to identify trends and patterns.
Use software programs specifically designed for agricultural analysis.
Engage experts to interpret complex data sets effectively.
Data Sharing and Collaboration
Encourage collaboration by sharing data with stakeholders.
Collaboration fosters innovation and problem-solving strategies.
Consider participating in local agricultural data networks.
Implementing Data-driven Decisions
Use insights gained from data to make strategic decisions.
Adjust farming practices based on data findings for better outcomes.
Document changes to assess their impact on soil health and yield.
Additional Resources
USDA Science and Research Strategy, 2023-2026: Cultivating …
Regenerative Agriculture: Good for Soil Health, but Limited Potential …